讲座题目:A unified framework towards complementary and explainable application of model and machine learning based methods to fault detection in automatic control systems
讲座时间:2022/07/22(周五) 14:00-16:00
腾讯会议ID:143-685-171
会议链接:https://meeting.tencent.com/dm/HINRZxZU4Q3R
直播地址:https://meeting.tencent.com/l/aexdn69sF7TF
邀请人:雷亚国 教授
讲座人:Steven X. Ding 教授
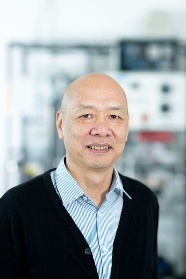
Steven X. Ding is a professor of control engineering and the head of the Institute of Automatic Control and Complex Systems (AKS) in the Faculty of Engineering at the University of Duisburg-Essen, Germany. He received the Ph.D. degree in the electrical engineering from the University of Duisburg in 1992. Between 1992 and 1994, he was with company Rheinmetall GmbH, Germany. From 1995 to 2001, he was a professor of control engineering at the University of Applied Science Lausitz in Senftenberg, Germany, and served as the vice president of this university during 1998 – 2000. Since 2001, he has been a full-professor of control engineering at the University of Duisburg-Essen. Prof. Ding is an independent researcher. His research interests are model-based and data-driven fault diagnosis, process monitoring and control as well as their applications to automotive and process industry, renewable energy, smart building systems as well as secure cyber-physical systems.
讲座简介:
Application of model- and observer-based methods to fault detection in automatic control systems is state of the art, although machine learning (ML) algorithms build the mainstream in research domain in recent years. A critical and obvious barrier for a wide and successful application of ML-based methods to industrial automatic control systems is their (application) explainability and limited capability of dealing with system dynamics. In the context of representation learning, the so-called latent (hidden) variable plays an important role. It has been recognised that an optimal reconstruction of process variables towards optimal fault detection is achieved, when the latent variable is a minimal sufficient statistic. In this talk, a newly established fault detection scheme, the projection-based fault detection framework, is first introduced. In this framework, both model- and ML-based fault detection methods can be complementarily applied to fault detection in dynamic systems. In particular, it provides us with a clear explanation and interpretation of latent variables and leads to successful applications of the ML-based methods to fault detection in automatic control systems with optimal detection performance.
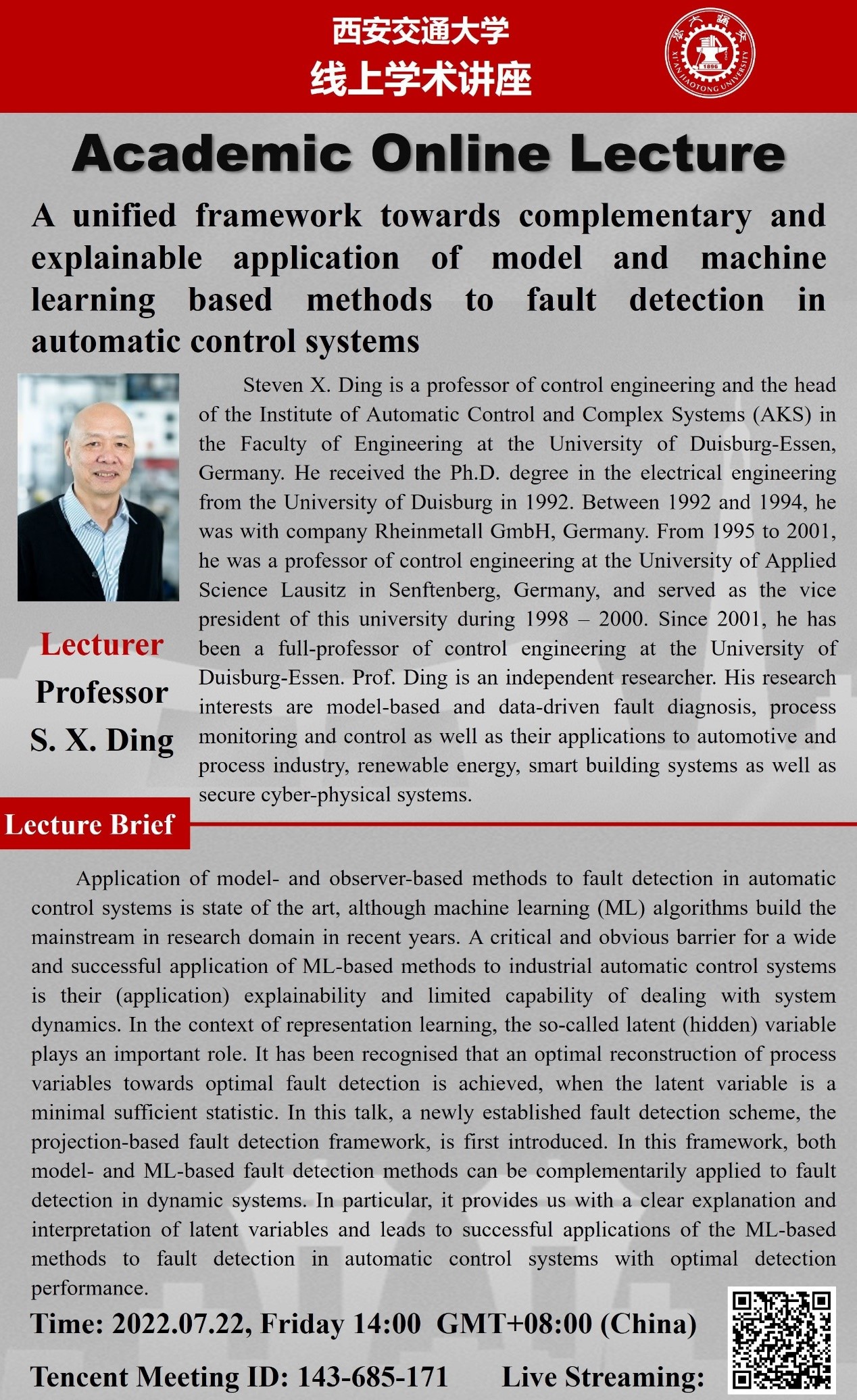